eDiscovery Daily Blog
eDiscovery Best Practices: For Successful Predictive Coding, Start Randomly
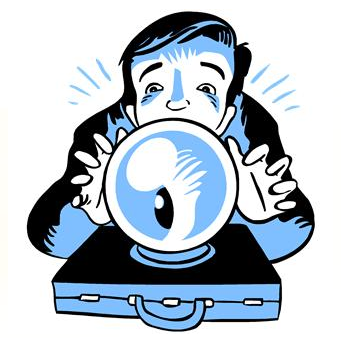
Predictive coding is the hot eDiscovery topic of 2012, with three significant cases (Da Silva Moore v. Publicis Groupe, Global Aerospace v. Landow Aviation and Kleen Products v. Packaging Corp. of America) either approving or considering the use of predictive coding for eDiscovery. So, how should your organization begin when preparing a collection for predictive coding discovery? For best results, start randomly.
If that statement seems odd, let me explain.
Predictive coding is the use of machine learning technologies to categorize an entire collection of documents as responsive or non-responsive, based on human review of only a subset of the document collection. That subset of the collection is often referred to as the “seed” set of documents. How the seed set of documents is derived is important to the success of the predictive coding effort.
Random Sampling, It’s Not Just for Searching
When we ran our series of posts (available here, here and here) that discussed the best practices for random sampling to test search results, it’s important to note that searching is not the only eDiscovery activity where sampling a set of documents is a good practice. It’s also a vitally important step for deriving that seed set of documents upon which the predictive coding software learning decisions will be made. As is the case with any random sampling methodology, you have to begin by determining the appropriate sample size to represent the collection, based on your desired confidence level and an acceptable margin of error (as noted here). To ensure that the sample is a proper representative sample of the collection, you must ensure that the sample is performed from the entire collection to be predictively coded.
Given the debate in the above cases regarding the acceptability of the proposed predictive coding approaches (especially Da Silva Moore), it’s important to be prepared to defend your predictive coding approach and conducting a random sample to generate the seed documents is a key step to defensibility of that approach.
Then, once the sample is generated, the next key to success is the use of a subject matter expert (SME) to make responsiveness determinations. And, it’s important to conduct a sample (there’s that word again!) of the result set after the predictive coding process to determine whether the process achieved a sufficient quality in automatically coding the remainder of the collection.
So, what do you think? Do you start your predictive coding efforts “randomly”? You should. Please share any comments you might have or if you’d like to know more about a particular topic.
Disclaimer: The views represented herein are exclusively the views of the author, and do not necessarily represent the views held by CloudNine Discovery. eDiscoveryDaily is made available by CloudNine Discovery solely for educational purposes to provide general information about general eDiscovery principles and not to provide specific legal advice applicable to any particular circumstance. eDiscoveryDaily should not be used as a substitute for competent legal advice from a lawyer you have retained and who has agreed to represent you.