eDiscovery Daily Blog
eDiscovery Trends: What the Heck is “Predictive Coding”?
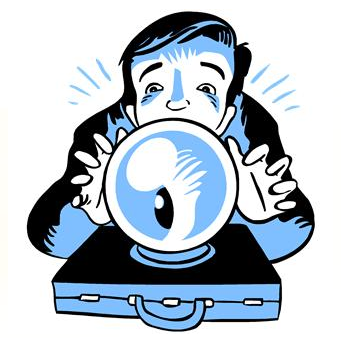
Yesterday, ALM hosted another Virtual LegalTech online "live" day online. Every quarter, theVirtual LegalTech site has a “live” day with educational sessions from 9 AM to 5 PM ET, most of which provide CLE credit in certain states (New York, California, Florida, and Illinois).
One of yesterday’s sessions was Frontiers of E-Discovery: What Lawyers Need to Know About “Predictive Coding”. The speakers for this session were:
Jason Baron: Director of Litigation for the National Archives and Records Administration, a founding co-coordinator of the National Institute of Standards and Technology’s Text Retrieval Conference (“TREC”) legal track and co-chair and editor-in-chief for various working groups for The Sedona Conference®;
Maura Grossman: Counsel at Wachtell, Lipton, Rosen & Katz, co-chair of the eDiscovery Working Group advising the New York State Unified Court System and coordinator of the 2010 TREC legal track; and
Bennett Borden: co-chair of the e-Discovery and Information Governance Section at Williams Mullen and member of Working Group I of The Sedona Conference on Electronic Document Retention and Production, as well as the Cloud Computing Drafting Group.
This highly qualified panel discussed a number of topics related to predictive coding, including practical applications of predictive coding technologies and results of the TREC 2010 Legal Track Learning Task on the effectiveness of “Predictive Coding” technologies.
Before discussing the strategies for using predictive coding technologies and the results of the TREC study, it’s important to understand what predictive coding is. The panel gave the best descriptive definition that I’ve seen yet for predictive coding, as follows:
“The use of machine learning technologies to categorize an entire collection of documents as responsive or non-responsive, based on human review of only a subset of the document collection. These technologies typically rank the documents from most to least likely to be responsive to a specific information request. This ranking can then be used to “cut” or partition the documents into one or more categories, such as potentially responsive or not, in need of further review or not, etc.”
The panel used an analogy for predictive coding by relating it to spam filters that review and classify email and learn based on previous classifications which emails can be considered “spam”. Just as no spam filter perfectly classifies all emails as spam or legitimate, predictive coding does not perfectly identify all relevant documents. However, they can “learn” to identify most of the relevant documents based on one of two “learning” methods:
- Supervised Learning: a human chooses a set of “exemplar” documents that feed the system and enable it to rank the remaining documents in the collection based on their similarity to the exemplars (e.g., “more like this”);
- Active Learning: the system chooses the exemplars on which human reviewers make relevancy determinations, then the system learns from those classifications to apply to the remaining documents in the collection.
Tomorrow, I “predict” we will get into the strategies and the results of the TREC study. You can check out a replay of the session at theVirtual LegalTech site. You’ll need to register – it’s free – then login and go to the CLE Center Auditorium upon entering the site (which is up all year, not just on "live days"). Scroll down until you see this session and then click on “Attend Now” to view the replay presentation. You can also go to the Resource Center at the site and download the slides for the presentation.
So, what do you think? Do you have experience with predictive coding? Please share any comments you might have or if you’d like to know more about a particular topic.