eDiscovery Daily Blog
A Technical Explanation of Near-Dupes – eDiscovery Tutorial
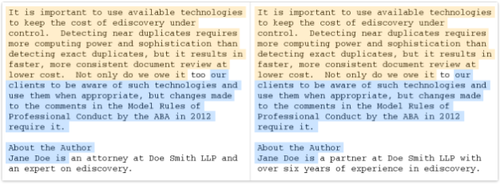
Bill Dimm provides a comprehensive and interesting description of near-dupes and the algorithms used to identify them in his Clustify blog (What is a near-dupe, really?). If you want to understand the “three reasonable, but different, ways of defining the near-dupe similarity between two documents”, bring your brain and check it out.
As we discussed last month, just because information volume in most organizations doubles every 18-24 months doesn’t mean that it’s all original. When reviewers are reviewing the same data again and again, it’s unnecessarily expensive and prone to mistakes.
As Bill notes in his post, “Near-duplicates are documents that are nearly, but not exactly, the same. They could be different revisions of a memo where a few typos were fixed or a few sentences were added. They could be an original email and a reply that quotes the original and adds a few sentences. They could be a Microsoft Word document and a printout of the same document that was scanned and OCRed with a few words not matching due to OCR errors.” I also classify examples such as a Word document published to an Adobe PDF file (where the content is the same, but the file format is different, so the hash value will be different) as near-duplicates because they won’t be de-duped with an MD5 or SHA-1 hash algorithm at the file level. You need an algorithm that looks for similarity in the document content.
Identifying near-duplicates that contain almost the same information reduces redundant review and saves costs. A recent client of mine had over 800,000 emails belonging to near-duplicate groupings that would have been impossible to identify without an effective algorithm to group them together.
Bill’s blog post goes on to discuss different methods for measuring similarity using mechanisms like a Jaccard index and a MinHash algorithm which counts shingles (don’t worry, they’re neither painful nor scaly). Understanding how your near-dupe software works is important. As Bill notes, “If misunderstandings about how the algorithm works cause the similarity values generated by the software to be higher than you expected when you chose the similarity threshold, you risk tagging near-dupes of non-responsive documents incorrectly (grouped documents are not as similar as you expected). If the similarity values are lower than you expected when you chose the threshold, you risk failing to group some highly similar documents together, which leads to less efficient review (extra groups to review).” His post is an excellent primer to developing that understanding.
So, what do you think? Do you have a plan for handling near-duplicates in your collection? Please share any comments you might have or if you’d like to know more about a particular topic.
Disclaimer: The views represented herein are exclusively the views of the author, and do not necessarily represent the views held by CloudNine Discovery. eDiscoveryDaily is made available by CloudNine Discovery solely for educational purposes to provide general information about general eDiscovery principles and not to provide specific legal advice applicable to any particular circumstance. eDiscoveryDaily should not be used as a substitute for competent legal advice from a lawyer you have retained and who has agreed to represent you.